No narrative, just short reports of things I’ve read, or listened to
Catch up service, conspiracy theory track:
RP#45 Radiation-laced chapatis as a test case for defining Conspiracy Theories
RP#44 Conspiracy Theories are propaganda for deep beliefs
RP#42 What conspiracy theorists get right
RP#29 Conspiracy thinking
EXPERIMENT REPORT: Cognitive biases: Mistakes or missing stakes?
New evidence that some biases are strongly “built in” to our thinking, not just due to laziness.
Offering people a month’s wages to get the questions right doesn’t effect four classic cognitive biases (base-rate neglect, anchoring, failure of contingent thinking, and intuitive reasoning), although it does make people try harder.
Abstract: Despite decades of research on heuristics and biases, evidence on the effect of large incentives on cognitive biases is scant. We test the effect of incentives on four widely documented biases: base-rate neglect, anchoring, failure of contingent thinking, and intuitive reasoning. In laboratory experiments with 1,236 college students in Nairobi, we implement three incentive levels: no incentives, standard lab payments, and very high incentives. We find that very high stakes increase response times by 40% but improve performance only very mildly or not at all. In none of the tasks do very high stakes come close to debiasing participants.
Enke, B., Gneezy, U., Hall, B., Martin, D., Nelidov, V., Offerman, T., & Van De Ven, J. (2023). Cognitive biases: Mistakes or missing stakes?. Review of Economics and Statistics, 1-45.
TALK: The Jordan Peterson Phenomenon: Nikos Sotirakopoulos
This talk (25 minutes, plus questions) considers both the thought of JBP and the intense reactions he evokes.
PREPRINT: The meaning of ‘reasonable’: Evidence from a corpus-linguistic study
What is a reasonable person anyway? Does it just mean the typical person, or does it imply the good, upright, citizen? Baumgartner & Kneer analyse tens of thousands of Reddit comments which use ‘coordinating conjunctions’ such as “What a cruel and sad world!”. From this, they can analyse which words ‘reasonable’ is used with, and hence what people mean when they say it.
ABSTRACT
The reasonable person standard is key to both Criminal Law and Torts. What does and does not count as reasonable behavior and decision-making is frequently deter-mined by lay jurors. Hence, laypeople’s understanding of the term must be considered, especially whether they use it predominately in an evaluative fashion. In this corpus study based on supervised machine learning models, we investigate whether laypeople use the expression ‘reasonable’ mainly as a descriptive, an evaluative, or merely a value-associated term. We find that ‘reasonable’ is predicted to be an evaluative term in the majority of cases. This supports prescriptive accounts, and challenges descriptive and hybrid accounts of the term—at least given the way we operationalize the latter. Interestingly, other expressions often used interchangeably in jury instructions (e.g. ‘careful,’ ‘ordinary,’ ‘prudent,’ etc), however, are predicted to be descriptive. This indicates a discrepancy between the intended use of the term ‘reasonable’ and the understanding lay jurors might bring into the court room.
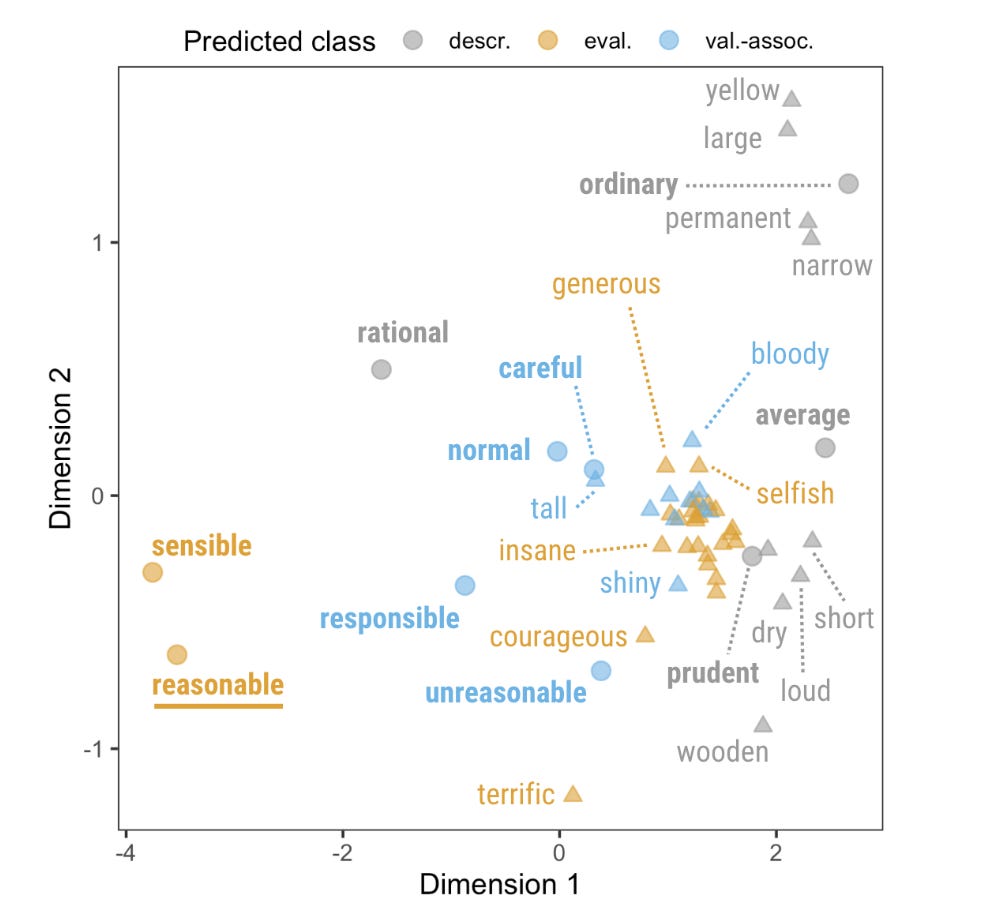
From the conclusion
both ‘rational’ and ‘reasonable’ are ordinarily associated with being sensible, intelligent, and logical, but they nevertheless carry very distinct connotations. While ‘rational’ generally refers to self- interest and agency, ‘reasonable’ is applied in cases where people are socially minded and caring. These differences in connotations could lead to different adjectival co-occurrences, which ultimately affect their sentiment dispersion. Our findings are also consistent with recent interesting results by Jaeger (2020), which suggest that the lay understanding of ‘reasonable’ differs considerably from economic rationality proposed by scholars of the Law & Economics tradition.
Baumgartner, L., & Kneer, M. (2023, August 25). The meaning of ‘reasonable’: Evidence from a corpus-linguistic study. https://doi.org/10.31234/osf.io/bt9c4. Forthcoming in Tobia, K. P. (Ed.). The Cambridge Handbook of Experimental Jurisprudence. Cambridge University Press.
PREPRINT: The wisdom of extremized crowds: Promoting erroneous divergent opinions increases collective accuracy
Really nice paper which combines a classic bias from the judgement and decision making literature and shows how it can be used to enhance accuracy on collective estimation/forecasting tasks (based on a simple mathematical model, across multiple experiments, using preregistration to confirm predictions - these are some of my favourite things).
The key idea is that the “wisdom of crowds” effect is enhanced when the accuracy of the individuals contributing judgements is enhanced OR the diversity of the individual judgements is enhanced. Lots of literature tests the first idea, this study tests the second. They induce enhanced diversity of judgements by using the anchoring effect to bias half of the participants to produce high estimate, and half to produce low estimates. As predicted, this makes the collective diversity (i.e. variance) of the judgements go up, but the collective error go down (i.e. the accuracy increased when you average out everyone’s answers). Neat!
Here’s the results figure for their final experiment, which asked a true forecasting question, something to which the answer was not known at the time. Green is the control group, purple is the group with the added bias, the “extemized crowd”:
I’m still pondering the mechanisms of this and the maths of their account. My understanding of the wisdom of crowds is that it works to the extent that individual judgements have a component driven by the true value (which is correlated across individuals) and a component driven by extraneous factors (which is not correlated across individuals). When you average over the crowd you keep the true value component and the extraneous factor component cancels out. Does this study show that the anchoring effect works by interacting with ‘extraneous’ components in our judgement process, without combining with whatever is driving the component based on the true value? What does that mean, in terms of cognitive mechanism? I’ll let you know if I make any progress on these questions
ABSTRACT:
The aggregation of many lay judgements can generate surprisingly accurate estimates. This effect, known as the “wisdom of the crowd”, has been demonstrated in domains such as medical decision-making, fact-checking news, and financial forecasting. Therefore, understanding the conditions that enhance the wisdom of the crowd has become a crucial issue in the social and behavioral sciences. Previous theoretical research identified two key factors driving this effect: the accuracy of individuals and the diversity of their opinions. Most available strategies to enhance the wisdom of the crowd have exclusively focused on improving individual accuracy while neglecting the potential of increasing opinion diversity. Here, we study a complementary approach to reduce collective error by promoting divergent and extreme opinions, using a cognitive bias called the “anchoring effect”. This method proposes to anchor half of the crowd to an extremely small value and the other half to an extremely large value before eliciting and averaging their estimates. As predicted by our mathematical modeling, three behavioral experiments demonstrate that this strategy concurrently increases individual error, opinion diversity, and collectively accuracy. Most remarkably, we show that this approach works even in a forecasting task where the experimenters did not know the correct answer at the time of testing. Overall, these results not only provide practitioners with a new strategy to forecast and estimate variables but also have strong theoretical implications on the epistemic value of collective decision-making.
Barrera-Lemarchand, F., Balenzuela, P., Bahrami, B., Deroy, O., & Navajas, J. (2023, August 14). The wisdom of extremized crowds: Promoting erroneous divergent opinions increases collective accuracy. https://doi.org/10.31234/osf.io/wvfm3
And finally…
END
Comments? Feedback? Fragments of reason? I am tom@idiolect.org.uk and on Mastodon at @tomstafford@mastodon.online